Correlational Designs 11 v4 Principles of Research in Behavioral
Table Of Content
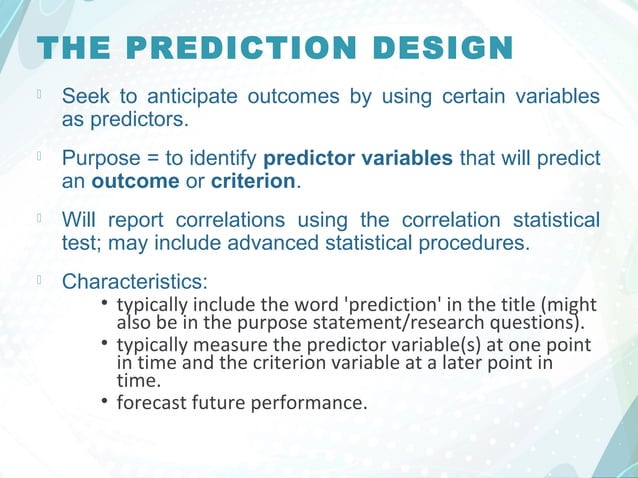
Regression analysis is a statistical method used to model the relationship between two or more variables. Researchers use regression analysis to predict the value of one variable based on the value of another variable. Regression analysis can help identify the strength and direction of the relationship between variables, as well as the degree to which one variable can be used to predict the other. Correlational and experimental research both use quantitative methods to investigate relationships between variables. But there are important differences in how data is collected and the types of conclusions you can draw.
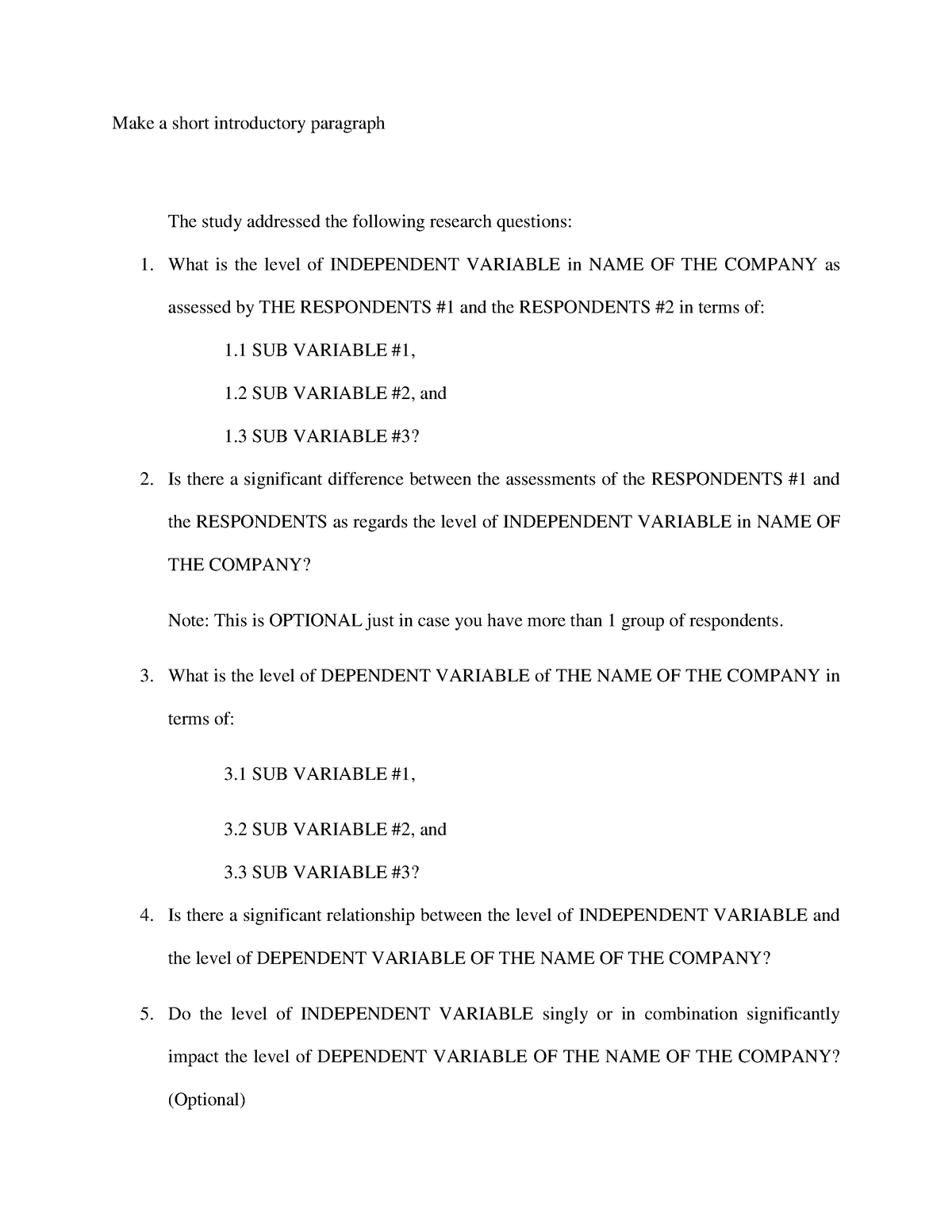
Statistical Significance
For example, a researcher might evaluate the validity of a brief extraversion test by administering it to a large group of participants along with a longer extraversion test that has already been shown to be valid. This researcher might then check to see whether participants’ scores on the brief test are strongly correlated with their scores on the longer one. Neither test score is thought to cause the other, so there is no independent variable to manipulate.
What are the Types of Correlational Research?
Linear and nonlinear correlation estimators unveil undescribed taxa interactions in microbiome data - Nature.com
Linear and nonlinear correlation estimators unveil undescribed taxa interactions in microbiome data.
Posted: Tue, 23 Aug 2022 07:00:00 GMT [source]
Perhaps being stressed has a negative effect on people’s ability to plan ahead (the directionality problem). Or perhaps people who are more conscientious are more likely to make to-do lists and less likely to be stressed (the third-variable problem). The crucial point is that what defines a study as experimental or correlational is not the variables being studied, nor whether the variables are quantitative or categorical, nor the type of graph or statistics used to analyze the data. Figure 7.2 shows data from a hypothetical study on the relationship between whether people make a daily list of things to do (a “to-do list”) and stress. Notice that it is unclear whether this design is an experiment or a correlational study because it is unclear whether the independent variable was manipulated.
4.2. Cross-sectional Analysis of EHR Documentation and Care Quality
Researchers are expected to demonstrate the interrater reliability of their coding procedure by having multiple raters code the same behaviors independently and then showing that the different observers are in close agreement. Kraut and Johnston, for example, video recorded a subset of their participants’ reactions and had two observers independently code them. The two observers showed that they agreed on the reactions that were exhibited 97% of the time, indicating good interrater reliability. Overall, the purpose of correlational research is to provide insight into the relationship between variables, which can be used to inform further research, interventions, or policy decisions. Factor analysis is a statistical method used to identify patterns among variables.
You might statistically control for these variables, but you can’t say for certain that lower working hours reduce stress because other variables may complicate the relationship. Different types of correlation coefficients and regression analyses are appropriate for your data based on their levels of measurement and distributions. This method often involves recording, counting, describing, and categorising actions and events.
This type of research is descriptive, unlike experimental research that relies entirely on scientific methodology and hypothesis. For example, correlational research may reveal the statistical relationship between high-income earners and relocation; that is, the more people earn, the more likely they are to relocate or not. A general limitation of a correlational study is that it can determine association between exposure and outcomes but cannot predict causation. The more specific limitations of the three case examples cited by the authors are listed below. Correlational studies, better known as observational studies in epidemiology, are used to examine event exposure, disease prevalence and risk factors in a population (Elwood, 2007). In eHealth, the exposure typically refers to the use of an eHealth system by a population of subjects in a given setting.
Imagine, for example, that a researcher administers the Rosenberg Self-Esteem Scale to 50 American college students and 50 Japanese college students. Although this “feels” like a between-subjects experiment, it is a correlational study because the researcher did not manipulate the students’ nationalities. The same is true of the study by Cacioppo and Petty comparing college faculty and factory workers in terms of their need for cognition. It is a correlational study because the researchers did not manipulate the participants’ occupations.
Characteristics of a Correlational Study
Three case examples were included to show how eHealth correlational studies are done. A negative correlation occurs when one variable increases while the other decreases. This means that as one variable increases, the other variable tends to decrease. For example, there is a negative correlation between the number of hours spent watching TV and physical activity level.
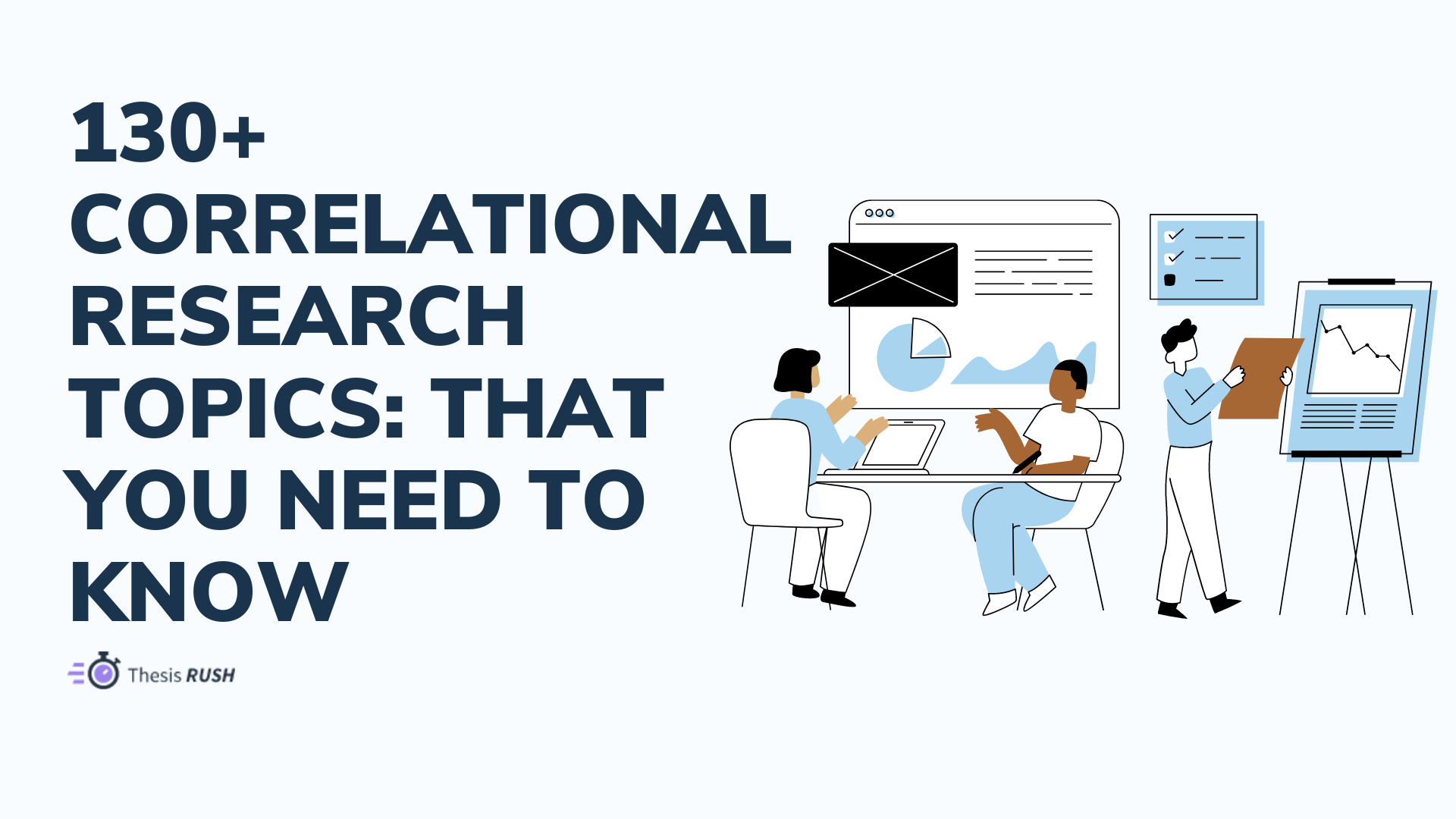
But if it was a correlational study, it could only be concluded that these variables are related. A common misconception among beginning researchers is that correlational research must involve two quantitative variables, such as scores on two extraversion tests or the number of daily hassles and number of symptoms people have experienced. However, the defining feature of correlational research is that the two variables are measured—neither one is manipulated—and this is true regardless of whether the variables are quantitative or categorical.
Correlational research is non-experimental as it does not involve manipulating variables using a scientific methodology in order to agree or disagree with a hypothesis. In correlational research, the researcher simply observes and measures the natural relationship between 2 variables; without subjecting either of the variables to external conditioning. For this method of correlational research, the research makes use of earlier studies conducted by other researchers or the historical records of the variables being analyzed. This method helps a researcher to track already determined statistical patterns of the variables or subjects. There are growing populations with multiple chronic conditions and healthcare interventions.
This information can be useful for predicting and explaining behavior, and for identifying potential risk factors or areas for intervention. Meta-analysis involves combining and analyzing the results of multiple studies to explore the relationships between variables across different contexts and populations. Meta-analysis is useful for identifying patterns and inconsistencies in the literature and can provide insights into the strength and direction of relationships between variables. A correlational study is a type of research design that looks at the relationships between two or more variables.
Archival data is a type of correlational research method that involves making use of already gathered information about the variables in correlational research. Since this method involves using data that is already gathered and analyzed, it is usually straight to the point. The four items specific to study design relate to the reporting of participants, statistical methods, descriptive results and outcome data.
The correlation coefficient is a numerical measure that quantifies the strength and direction of the relationship between two variables. There are different types of correlation coefficients, including Pearson's correlation coefficient (for linear relationships), Spearman's rank correlation coefficient (for ordinal data), and Kendall's tau (for non-parametric data). Here, we'll focus on calculating Pearson's correlation coefficient (r), which is commonly used for interval or ratio-level data. It is important to note that a correlation coefficient only reflects the linear relationship between 2 variables; it does not capture non-linear relationships and cannot separate dependent and independent variables. The correlation coefficient helps you to determine the degree of statistical relationship that exists between variables.
In medicine, correlational research can be used to investigate the relationship between lifestyle factors and disease outcomes. Path analysis is a statistical method used to model the relationship between multiple variables. Researchers use path analysis to test causal models and identify direct and indirect effects between variables. You've probably heard the phrase, "correlation does not equal causation." This means that while correlational research can suggest that there is a relationship between two variables, it cannot prove that one variable will change another. When reviewing old research, little information might be available about who conducted the research, how a study was designed, who participated in the research, as well as how data was collected and interpreted.
Comments
Post a Comment